Introduction to AI in Agriculture
In recent years, Artificial Intelligence (AI) has made significant strides in transforming multiple industries, with agriculture being no exception. Integrating AI into agriculture, often termed as Smart Farming, is shifting traditional farming methods towards more efficient, data-driven practices. This transition is critical for addressing pressing challenges like increasing food demand, labor shortages, and environmental sustainability.
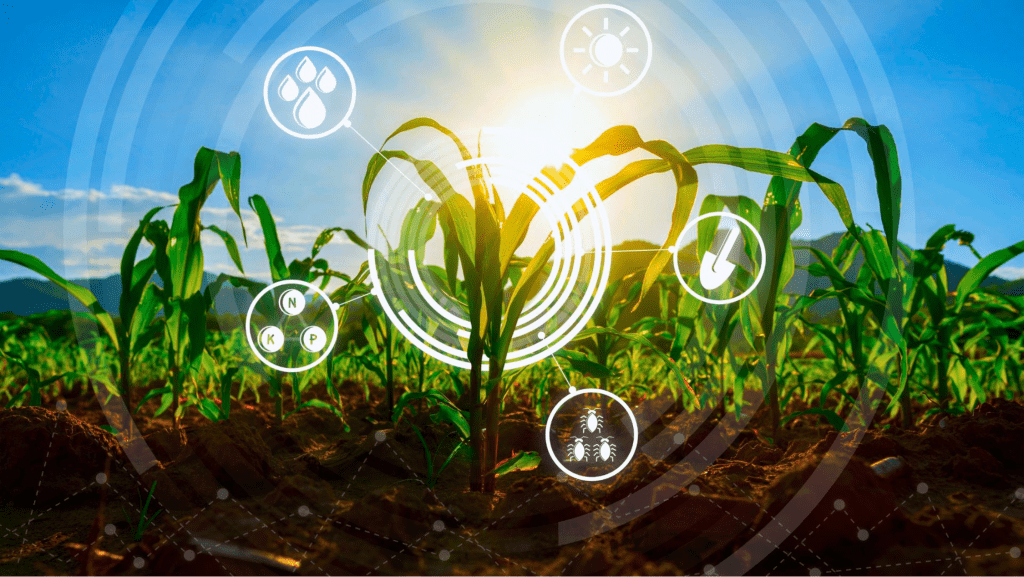
Key Applications of AI in Agriculture
Precision Agriculture: Enhancing Efficiency
Precision Agriculture uses AI-driven data analytics to monitor crops at a granular level. Through satellite imagery, drone technology, and sensors, farmers receive detailed information about soil health, moisture levels, and crop growth. This information enables targeted fertilization and water management, resulting in higher yields with fewer resources.
AI in Crop Monitoring and Disease Detection
One of AI’s most impactful uses in agriculture is its role in crop health monitoring. AI algorithms analyze images captured by drones or satellites to detect signs of crop stress, disease, or pest infestation. Early detection through machine learning models can lead to prompt interventions, preserving crop health and preventing yield losses.
Automated Harvesting: Reducing Labor Dependency
The development of AI-powered harvesters has revolutionized harvesting, especially for labor-intensive crops. These machines, equipped with computer vision and robotic arms, can pick fruits and vegetables with minimal human intervention, addressing labor shortages and boosting productivity.
Technologies Driving AI in Agriculture
Machine Learning Models for Predictive Analysis
Machine learning algorithms are core to AI-driven agriculture. These models analyze historical data and current conditions to forecast crop yields, weather patterns, and market prices. Such predictive capabilities allow farmers to make informed decisions on crop selection, harvesting times, and pricing strategies.
Computer Vision for Crop and Soil Analysis
Computer Vision technology helps in analyzing images from fields, providing insights into crop quality, soil conditions, and weed identification. This AI-driven analysis helps in minimizing manual inspections, allowing farmers to detect anomalies efficiently and focus on high-need areas.
IoT and Sensors for Real-Time Monitoring
Internet of Things (IoT) devices, when combined with AI, create a network of sensors that continuously monitor conditions like temperature, humidity, pH levels, and soil moisture. These sensors transmit real-time data, enabling immediate responses and adaptive farming practices to maximize crop health.
Benefits of AI in Agriculture
Optimizing Resource Management
AI-driven solutions in agriculture have proven essential for resource management. By analyzing field data, AI helps in conserving water, reducing pesticide usage, and lowering energy consumption, leading to sustainable practices that conserve natural resources.
Improving Yield Prediction
Accurate yield prediction models, powered by machine learning, provide forecasts based on real-time data and historical trends. This information allows farmers to plan and allocate resources efficiently, minimizing losses and ensuring better market prices.
Reducing Environmental Impact
AI promotes sustainable farming by optimizing resource use and minimizing waste. With precision agriculture, farms can reduce their carbon footprint, contributing to global environmental goals while also improving the health of their soils.
Challenges in Implementing AI in Agriculture
High Initial Costs and Accessibility
Implementing AI solutions often involves substantial upfront costs, including expenses for advanced equipment, software, and training. For small-scale farmers, this investment may be challenging, and without widespread access, there is a risk of creating a technology divide in the agriculture sector.
Data Privacy and Security
With the vast amount of data collected through sensors and devices, data privacy is a critical concern. Protecting farmers’ data against unauthorized access and ensuring that AI technology complies with data security standards is essential for its safe adoption.
Need for Skilled Workforce
Using AI in agriculture requires technical expertise to operate advanced tools and interpret data effectively. Therefore, training the agricultural workforce and providing access to educational resources are crucial steps for the successful integration of AI in this field.
Case Studies: Successful AI Implementation in Agriculture
Case Study 1: AI in Irrigation Management
A notable example of AI in agriculture is in irrigation management. In regions with water scarcity, AI-driven irrigation systems use real-time data to adjust water levels, ensuring crops receive adequate water without waste. This approach has resulted in significant water savings and improved crop quality in many regions.
Case Study 2: Pest Control through Predictive Analytics
Some farms are using AI to monitor pest activity and predict outbreaks. By analyzing weather patterns, crop conditions, and historical pest data, these systems provide early warnings, allowing farmers to take preventive measures and reduce pesticide use.
Future of AI in Agriculture
Autonomous Farming: A New Era of Robotics
With advancements in robotics, fully autonomous farms are becoming more plausible. Self-driving tractors, robotic weeders, and autonomous planters will reshape agriculture by enabling more efficient farm management and eliminating some labor-intensive tasks.
Integration with Climate-Resilient Agriculture
As climate change impacts crop yields, AI is expected to play a critical role in climate-resilient agriculture. By combining climate data with predictive models, AI can help farmers choose crop varieties better suited to changing conditions, ensuring stable food supplies.
AI-Powered Supply Chain Optimization
AI has the potential to revolutionize the agricultural supply chain. By tracking produce from farm to table, AI can improve logistics, reduce spoilage, and ensure that fresh produce reaches consumers faster. This technology may also help in balancing demand and supply, minimizing food waste on a larger scale.
Conclusion
AI’s role in agriculture is not just transformative but necessary for meeting the growing global food demands in an environmentally sustainable way. By optimizing resource use, improving crop yields, and automating labor-intensive tasks, AI empowers farmers to adopt smarter, more efficient farming practices. However, widespread adoption will depend on making this technology accessible and addressing challenges related to cost, data security, and skills development.
FAQs
How does AI help in reducing water usage in agriculture?
AI optimizes water usage by using data from sensors to adjust irrigation levels, ensuring crops receive adequate moisture without wastage. This approach is particularly effective in water-scarce regions.
What role does AI play in pest management?
AI in pest management involves using predictive analytics to anticipate pest outbreaks based on weather and crop data. Early warnings allow farmers to take preventive measures, reducing the need for pesticides.
Can small-scale farmers benefit from AI?
While AI has high initial costs, there are affordable AI-driven solutions, especially in precision agriculture. Many governments and organizations are also working to make AI tools accessible for small-scale farmers.
Is AI in agriculture environmentally friendly?
Yes, AI promotes environmentally friendly practices by reducing resource use and lowering greenhouse gas emissions through optimized farming methods, which aligns with sustainability goals.
What are the limitations of AI in agriculture?
Some challenges include high implementation costs, data privacy concerns, and the need for technical expertise to operate AI-driven systems effectively. Addressing these issues will be crucial for broader adoption.