Predictive analytics is not just a buzzword; it’s a transformative force driving the evolution of agriculture. From forecasting crop yields to optimizing resource allocation, this technology empowers farmers to make data-driven decisions. Let’s dive deep into how predictive analytics is reshaping farm management.
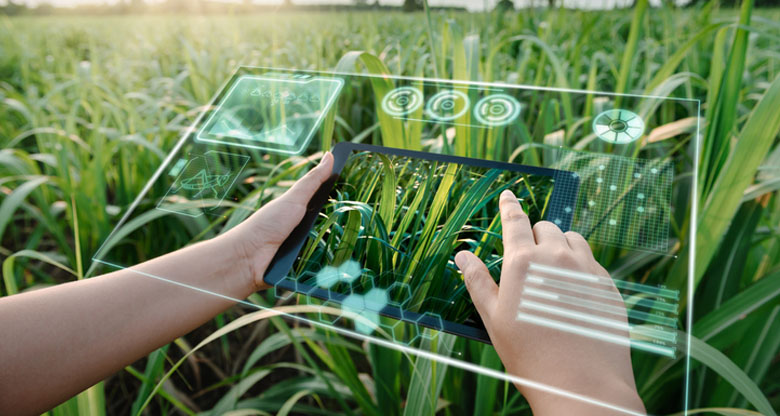
What is Predictive Analytics in Agriculture?
Predictive analytics uses data, statistical algorithms, and machine learning techniques to identify patterns and forecast future outcomes. In agriculture, it enables farmers to anticipate issues and capitalize on opportunities by analyzing weather, soil, crop, and market data.
Key Applications of Predictive Analytics in Farming
1. Forecasting Crop Yields
Predictive analytics tools process historical and real-time data to estimate potential yields. This enables farmers to plan logistics, manage inventory, and set accurate expectations for the harvest season.
2. Pest and Disease Management
Machine learning models analyze environmental and crop data to predict pest infestations or disease outbreaks, allowing preventive measures to be taken well in advance.
3. Weather Prediction and Risk Management
Advanced analytics tools interpret meteorological data to provide accurate weather forecasts, helping farmers make informed decisions on planting, irrigation, and harvesting.
4. Soil Health Monitoring
Predictive tools assess soil properties to recommend optimal fertilization and crop rotation strategies, ensuring long-term productivity.
5. Optimizing Resource Allocation
Data-driven insights guide efficient use of water, fertilizers, and energy, reducing waste and maximizing returns.
The Technologies Behind Predictive Analytics in Agriculture
Big Data and IoT Integration
Farmers collect massive amounts of data through IoT devices like sensors, drones, and GPS tools. This data forms the foundation for predictive analytics, ensuring accuracy and relevance.
Artificial Intelligence (AI) and Machine Learning
AI algorithms analyze patterns in agricultural data to deliver actionable insights. Machine learning continuously improves these predictions by adapting to new data inputs.
Geospatial Analytics
Satellite imagery and GPS data provide geospatial insights, enabling farmers to monitor land use and crop health with precision.
Cloud Computing
Cloud platforms enable real-time data processing and accessibility, ensuring seamless integration of predictive tools into farming operations.
The Benefits of Predictive Analytics for Farmers
1. Enhanced Decision-Making
With precise data at their fingertips, farmers can make informed choices about crop selection, planting schedules, and resource use.
2. Cost Reduction
Optimizing inputs such as water and fertilizers reduces expenses while maintaining high yields.
3. Risk Mitigation
Early warnings of pests, diseases, or adverse weather conditions allow proactive measures, minimizing potential losses.
4. Improved Sustainability
Predictive analytics promotes sustainable practices by reducing waste and optimizing resource usage.
Challenges in Adopting Predictive Analytics in Agriculture
1. High Initial Investment
The cost of acquiring IoT devices, sensors, and software can be a barrier for small-scale farmers.
2. Data Reliability
Accurate predictions depend on high-quality data, which may not always be available.
3. Technical Expertise
Many farmers lack the technical skills required to interpret and utilize predictive analytics tools effectively.
The Future of Predictive Analytics in Agriculture
As technology advances, predictive analytics will become more accessible and accurate. Integration with blockchain, AI advancements, and 5G connectivity will drive even greater adoption, ensuring every farmer, regardless of scale, can harness its benefits.
Conclusion
Predictive analytics is not just transforming agriculture—it’s revolutionizing it. By leveraging data and cutting-edge technology, farmers can anticipate challenges, optimize resources, and increase profitability. As the world grapples with climate change and a growing population, predictive analytics offers a beacon of hope for sustainable and efficient farming.
FAQs
1. What is predictive analytics in agriculture?
Predictive analytics involves using data and algorithms to forecast agricultural outcomes, helping farmers make informed decisions.
2. How does predictive analytics help in crop yield forecasting?
By analyzing historical and real-time data, predictive tools estimate potential yields, enabling better resource and logistics planning.
3. What technologies power predictive analytics in farming?
Key technologies include IoT, AI, machine learning, geospatial analytics, and cloud computing.
4. What are the challenges in adopting predictive analytics?
Challenges include high initial costs, data reliability issues, and the need for technical expertise.
5. Is predictive analytics sustainable for farming?
Yes, predictive analytics promotes sustainability by optimizing resource use, reducing waste, and ensuring efficient farming practices.